In a groundbreaking development, Google DeepMind has unveiled AlphaGeometry2, an advanced artificial intelligence system that has outperformed the average gold medalist in the prestigious International Mathematical Olympiad (IMO). This achievement marks a significant milestone in AI’s evolving capabilities, particularly in tackling complex mathematical problems that require deep reasoning and logical deduction.
The Rise of AlphaGeometry2: A New Benchmark in AI Mathematics
AlphaGeometry2 builds upon its predecessor, AlphaGeometry, which DeepMind introduced last January. The latest iteration showcases remarkable improvements, successfully solving 84% of geometry problems from the last 25 years of IMO competitions. Given that IMO is widely regarded as the most challenging math contest for high school students, this feat is no small accomplishment.
But why is DeepMind so invested in a high-school-level math competition? The answer lies in the broader implications of mathematical reasoning for AI development. Solving Euclidean geometry problems—where theorems and logical proofs play a fundamental role—demands a combination of abstract thinking, structured problem-solving, and pattern recognition. If AI can master these challenges, it could pave the way for more sophisticated reasoning abilities applicable to a wide range of scientific and technological fields.
A Hybrid Approach to Problem-Solving
At the core of AlphaGeometry2’s success is its hybrid architecture, which combines a neural network-based language model with a symbolic reasoning engine. Specifically, it leverages a model from Google’s Gemini family of AI models, working alongside a symbolic engine that applies mathematical rules to infer logical solutions.
Olympiad geometry problems often require the introduction of additional constructs—such as points, lines, or circles—to arrive at a proof. The Gemini model predicts which constructs could be useful, while the symbolic engine verifies these steps for logical consistency. This dual approach allows AlphaGeometry2 to explore multiple solution paths simultaneously, increasing the likelihood of arriving at a valid proof.
To compensate for the scarcity of structured geometry training data, DeepMind generated an extensive synthetic dataset consisting of over 300 million theorems and proofs of varying complexity. This dataset provided the foundation for training the model to recognize patterns, infer relationships, and solve intricate problems with remarkable accuracy.
Outperforming Gold Medalists: The Numbers Speak
To assess AlphaGeometry2’s prowess, DeepMind selected 45 geometry problems from IMO competitions spanning 2000 to 2024. Due to technical considerations, some problems were split, resulting in a total of 50 problems. The AI system managed to solve 42 out of 50—surpassing the average gold medalist score of 40.9. This firmly establishes AlphaGeometry2 as one of the most capable AI-driven mathematical problem solvers to date.
However, there are still limitations. AlphaGeometry2 struggles with problems involving a variable number of points, nonlinear equations, and inequalities. Additionally, while it excelled in solving past IMO problems, its performance dropped when confronted with 29 unpublished problems that had been nominated by mathematicians but never used in the actual competition. On this tougher problem set, it solved only 20 out of 29, highlighting areas for further improvement.
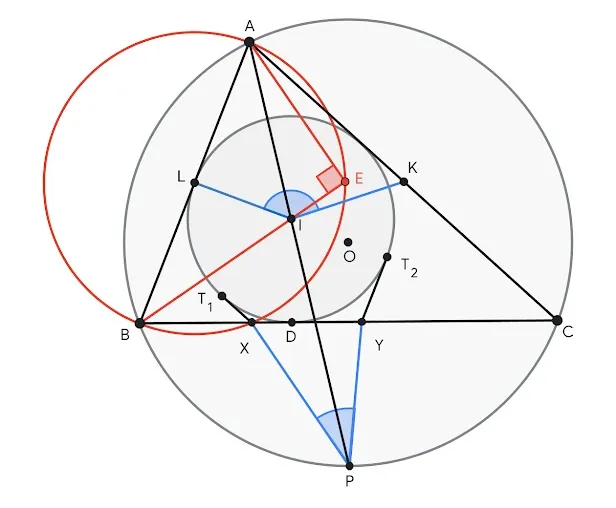
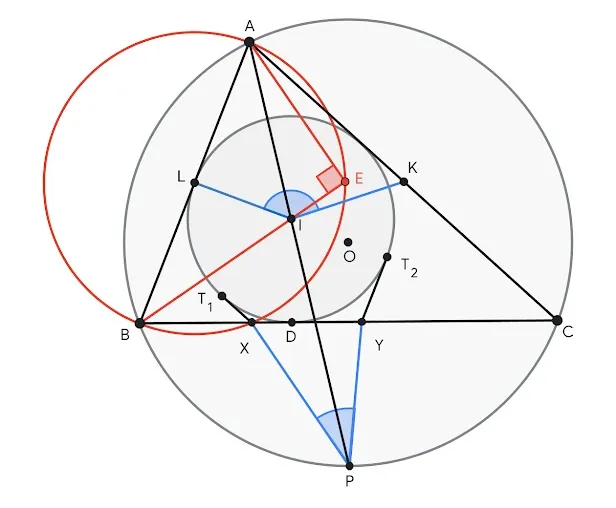
Symbolic AI vs. Neural Networks: A Continuing Debate
The success of AlphaGeometry2 fuels an ongoing debate in AI research: Should AI systems be based on symbolic reasoning (manipulating symbols using defined rules) or neural networks (which learn through statistical approximation and pattern recognition)?
Neural networks have powered major AI breakthroughs in areas like language processing, computer vision, and speech recognition. However, symbolic AI—often dismissed as outdated—has its own strengths. Unlike neural networks, symbolic AI can encode structured knowledge, follow explicit logical rules, and provide transparent explanations for its conclusions.
AlphaGeometry2 takes a middle path, integrating both symbolic reasoning and neural network-based inference. This hybrid approach seems to offer the best of both worlds: the flexibility and pattern-matching abilities of deep learning, coupled with the rigorous logical structure of symbolic AI.
Interestingly, DeepMind’s study found that o1, another AI model with a purely neural network-based architecture, failed to solve any of the IMO problems that AlphaGeometry2 successfully tackled. This suggests that symbolic AI still has a crucial role to play in advancing AI’s reasoning capabilities.
The Road Ahead: Can AI Become Self-Sufficient in Mathematics?
One intriguing finding from the research hints at the potential for future AI models to solve mathematical problems independently. Preliminary evidence suggests that AlphaGeometry2’s language model was capable of generating partial solutions without relying on its symbolic engine. While this is not yet enough to replace symbolic reasoning, it indicates a future where large language models might independently handle complex mathematical reasoning—provided their accuracy and efficiency improve.
DeepMind acknowledges that challenges remain, particularly in terms of model speed and the risk of hallucination (AI generating incorrect or misleading answers). Until these issues are resolved, symbolic engines will remain essential for ensuring reliability in AI-driven mathematical applications.
Conclusion: A Step Closer to Generalized AI Reasoning
AlphaGeometry2’s success in solving IMO-level geometry problems is more than just an academic milestone; it’s a glimpse into AI’s future potential. By combining deep learning with structured reasoning, DeepMind has taken a significant step toward AI systems capable of generalizable problem-solving across mathematics, engineering, and beyond.
As AI continues to evolve, the integration of symbolic reasoning and neural networks may prove to be the key to unlocking higher levels of intelligence. With further advancements, we may one day see AI systems that can rival not just IMO gold medalists, but even the greatest mathematical minds in history. Until then, AlphaGeometry2 stands as a testament to what’s possible when AI and mathematics converge.